What is Data Analytics?
The process of data analytics involves analyzing raw information to identify trends and find answers. The field is broad. This process can include many different goals and techniques that vary from industry to industry.
Data analytics can be used for a variety of purposes. Combining these elements can result in a successful data analytics initiative that answers questions about historical trends and future forecasts, as well as decision-making.
Moreover, data analytics can be descriptive, diagnostic, predictive, or prescriptive. Data summarization, analysis of cause and effect, prediction of future events, and suggestions for action are some of its objectives. This procedure is vital in industries like manufacturing, healthcare, marketing, and finance to improve operations and make more accurate judgments.
Role of AI in Data Analytics
AI is rapidly becoming widely accepted across many applications. It significantly enhances data analytics by providing predictive insights.
Artificial intelligence (AI) plays a crucial role in data analytics by automating data processing. It also reveals hidden patterns and delivers predicted insights into data. Large datasets may be accurately and effectively analyzed because of AI techniques and applications. Artificial Intelligence (AI) has the potential to enhance decision-making, customize the consumer experience, and optimize processes instantly.
AI Techniques in Data Analytics:
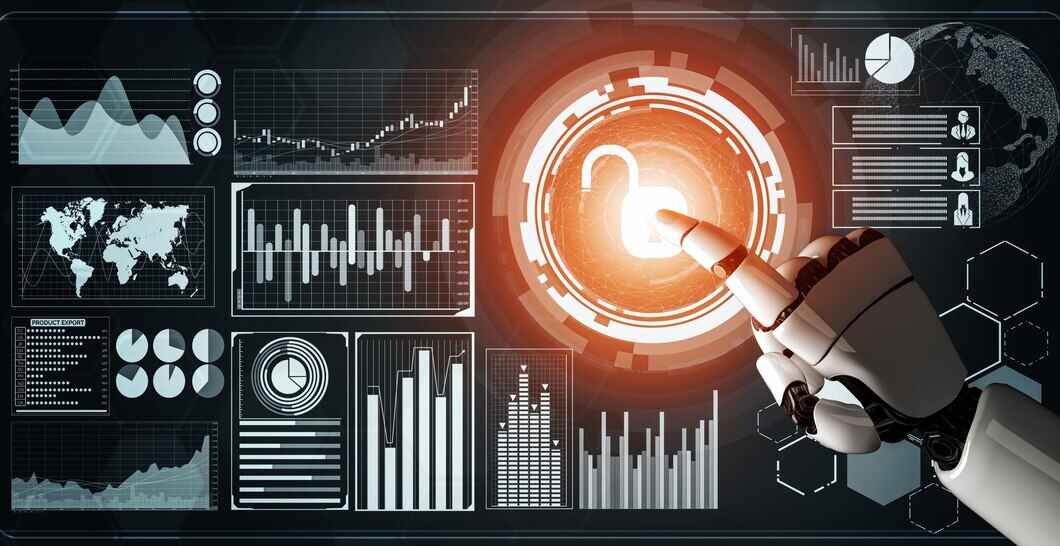
Machine Learning
Machine learning (ML) is a subfield of AI that aims to assist computers to learn more accurately by observing and imitating human behavior. It is a technique that uses historical data to identify patterns and trends and predict future outcomes.
Benefits of ML
Machine learning (ML) offers numerous benefits:
- By automating time-consuming tasks, and repetitive operations, machine learning algorithms allow humans to focus on higher-order, more creative work.
- It generates personalized experiences by studying the user's preferences and behavior using algorithms.
- Customers can get their questions answered quickly using chatbots, virtual assistants, and other ML-powered apps.
- Machine learning allows for real-time data study, which can lead to quick insights and actions.
- With machine learning, businesses may develop quickly and obtain a competitive advantage.
- By offering insights and recommendations based on data, ML improves decision support.
Natural Language Processing (NLP)
Natural language processing (NLP) is a branch of AI that focuses on teaching computers to comprehend and interact with human language via the use of machine learning. It enables computers to comprehend, identify, and generate written and spoken language.
Benefits of NLP
Here are some key benefits:
- Natural language processing (NLP) chatbots and virtual assistants can increase customer happiness by responding instantly to consumer questions.
- Natural language processing algorithms can identify whether a piece of text contains positive, neutral, or negative emotions.
- By enabling automatic translation, it eliminates linguistic barriers.
- Natural language processing (NLP) technology has improved the accuracy of text-to-speech conversions.
- Invoices, contracts, forms, and other similar documents can have their data extracted using this program.
- People with disabilities can greatly benefit from NLP. It offers services that convert text to speech and speech to text.
Deep Learning (DL)
Deep learning is a subset of machine learning that involves in imitating the brain's intricate decision-making process by employing multilayered neural networks; Convolutional Neural Networks (CNNs), Recurrent Neural Networks (RNNs), and Generative Adversarial Networks (GANs).
Distinct from machine learning, deep learning is characterized by the construction of artificial neural networks.
Benefits of DL
Following are a few of the key benefits of deep learning.
- Without the need for human feature engineers, deep learning models can autonomously gather useful features and information.
- When it comes to language processing, picture and speech recognition, and other similar tasks, deep neural networks and models can attain very high levels of accuracy.
- Data that is not organized, such as text, audio, or images, are ideal for DL's processing and analysis capabilities.
- By passing intermediate phases, the DL model can be trained end-to-end, allowing it to acquire the mapping of inputs to outputs immediately.
- Analyzing data and making decisions in real time is made possible by deep learning. Applications like autonomous driving, video surveillance, and fraud detection rely on this.
- Deep learning has been a game-changer in the field of artificial intelligence, expanding the capabilities of AI systems.
Applications of AI in Data Analytics
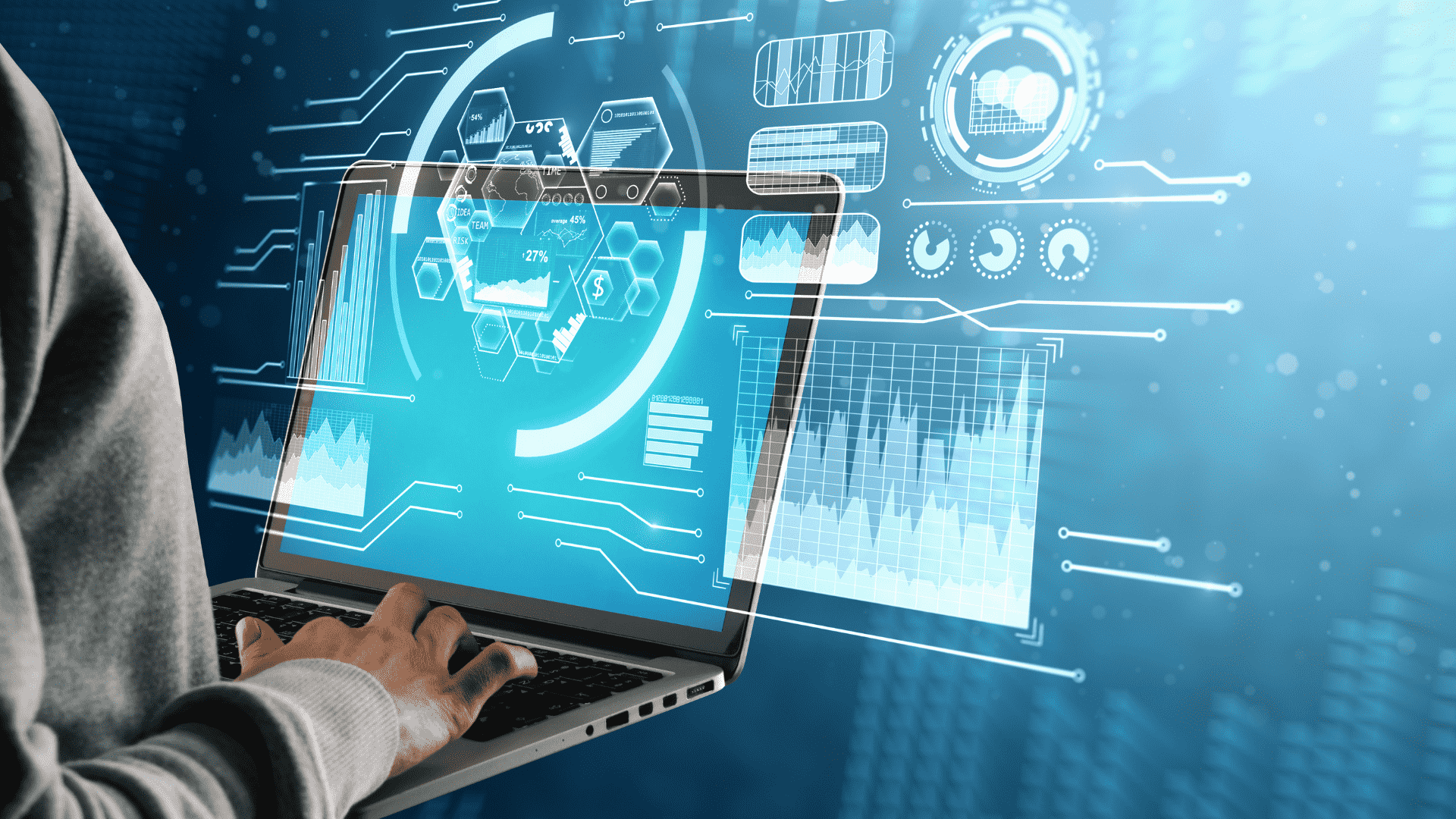
Automated Data Processing
Automated Data Processing refers to the use of AI to do challenging and frequently performed tasks that were previously done by humans. It typically covers gathering, cleaning, transforming, and integrating data from several sources. Large data sets may be processed quickly by AI algorithms, which guarantee consistency and accuracy while lowering the possibility of human error. Additionally, it improves data quality and sets it up for additional research and understanding.
Predictive Analytics
Predictive analytics examines past data to find trends and patterns by effectively using artificial intelligence (AI) algorithms. These can be utilized to forecast upcoming events and trends. Regression analysis, decision trees, and neural networks are a few examples of machine learning models that are trained on historical data to predict variables including risk factors, market patterns, and consumer behavior. By using such projections, businesses can minimize potential risks, streamline processes, and make informed choices.
Anomaly Detection
Anomaly detection is the process of identifying unusual patterns in data. AI models are skilled at figuring out a dataset's regular behavior and can identify anomalies that could be signs of fraud, malfunctioning technology, or security lapses. Neural networks, statistical techniques, and clustering are frequently employed for this purpose. Organizations can reduce the risk of losses by promptly responding to possible concerns through automation.
Real-Time Data Analysis
Artificial Intelligence (AI) is essential in providing real-time analytics. It uses quick and effective algorithms that can handle continuous data streams. This analysis is essential in situations like supply chain management, financial market monitoring, and e-commerce personalized recommendation systems. By giving immediate and pertinent information, real-time data analysis enables firms to adjust quickly to changing circumstances and enhance customer experiences.
Personalization
Artificial intelligence (AI) can be effectively used for personalization. It is used to adapt experiences, products, and services according to the preferences and habits of each user. By analyzing data like browsing history, user interactions, and purchase trends, AI models can predict what a customer is likely to want or need. This strategy increases consumer satisfaction and engagement by using tailored content, targeted advertising, and relevant advice.
Image and Video Analysis
Deep learning is a powerful AI method that can be used to extract useful information from visual images. Convolutional neural network (CNN) is a common technique to identify objects, scenes, and patterns in images and movies. This technology is used in a variety of industries including autonomous driving, security, and healthcare. AI-powered video and picture analysis can streamline many jobs, such as real-time virtual reality tours, security threat analyses on CCTV footage, and manufacturing fault detection.
Wrapping up
Artificial intelligence (AI) is crucial in data analytics because it can quickly and efficiently process large data sets to find predictive insights. Various AI techniques can detect trends, abnormalities, and connections that are difficult for human data analysts to see. AI can be used to improve productivity and streamline business processes. It gives businesses a competitive advantage in an increasingly data-driven world. This capability improves decision-making by generating more precise and knowledgeable outcomes.
Frequently Asked Questions
AI in data analytics offer various benefits. The key benefits include analysis of complex data, making accurate predictions, and faster data processing. Large datasets can also be handled with it along with deeper insights.
AI techniques contribute to data analysis by enabling complex data analysis, making accurate forecasts, and continually improving quality.
AI can enhance data analytics through automation, accuracy, real-time analysis and advanced predictive and prescription insights.
Yes, AI can efficiently analyze large datasets, revealing patterns and trends that would be impossible or difficult for humans to detect.